Mix2FLD: Downlink Federated Learning After Uplink Federated Distillation With Two-Way Mixup
- RAMO
- 2020년 6월 17일
- 1분 분량
In order to achieve both high accuracy and communication-efficiency under uplink-downlink asymmetric channels, we propose a distributed ML framework, dubbed Mix2FLD. As depicted below, Mix2FLD is built upon two key algorithms: federated learning after distillation (FLD) and Mixup data augmentation. Specifically, by leveraging FLD, each device in Mix2FLD uploads its local model outputs as in FD, and downloads the model parameters as in FL, thereby coping with the uplink-downlink channel asymmetry. In addition, Mix2FLD solves a model output-to-parameter conversion by utilizing Mix2up to collect additional training samples from devices while preserving data privacy.
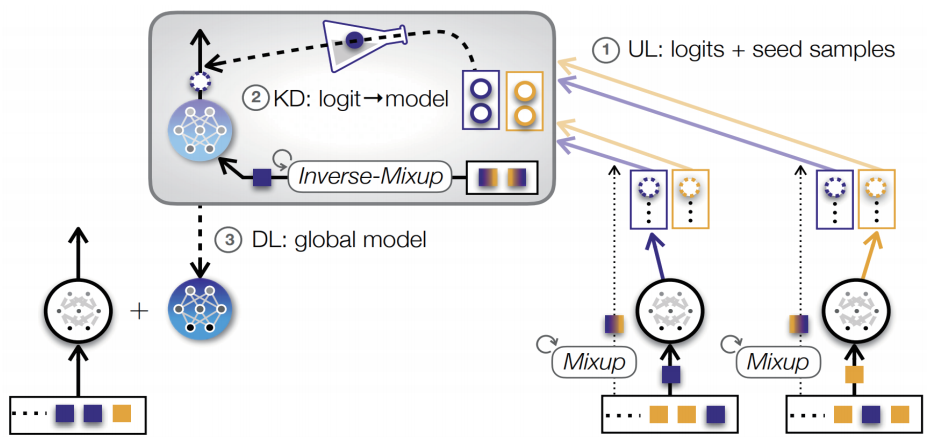
Abstract: This letter proposes a novel communication-efficient and privacy-preserving distributed machine learning framework, coined Mix2FLD. To address uplink-downlink capacity asymmetry, local model outputs are uploaded to a server in the uplink as in federated distillation (FD), whereas global model parameters are downloaded in the downlink as in federated learning (FL). This requires a model output-to-parameter conversion at the server, after collecting additional data samples from devices. To preserve privacy while not compromising accuracy, linearly mixed-up local samples are uploaded, and inversely mixed up across different devices at the server. Numerical evaluations show that Mix2FLD achieves up to 16.7% higher test accuracy while reducing convergence time by up to 18.8% under asymmetric uplink-downlink channels compared to FL.
Authors: Seungeun Oh, Jihong Park, Eunjeong Jeong, Hyesung Kim, Mehdi Bennis, and Seong-Lyun Kim, “Mix2FLD: Downlink Federated Learning After Uplink Federated Distillation With Two-Way Mixup,” accepted to IEEE Communications Letters, June 2020.
Commenti